Evaluation
A crowd flow generative model can be seen as both a classification as well as a regression problem.
Classification:
The classification problem can be defined as predicting the correct class for one unit of flow from a given origin. This task involves categorizing a single unit of flow based on its origin into predefined classes. For example, the objective may be to determine the next destination of an individual starting from a specific location. The output of this problem can be seen as more or less flow to a destination.
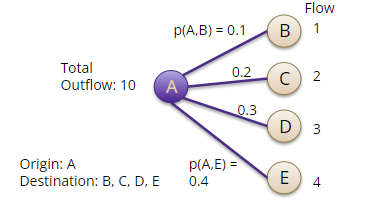
Regression:
The regression problem is defined as predicting the correct numeric flow for a directed tuple of locations. This task involves estimating the continuous numeric value representing the flow between a pair of locations. For instance, the objective may be to predict the number of people moving from location A to location B within a specific timeframe. The output of this problem is continuous numeric values indicating the magnitude of flow between locations.
For our fairness evaluation metrics, we consider two factors:
- Separation as a fundamental fairness criterion:
For classification problems, ‘Separation’ is recognized as a fundamental fairness criterion. Separation emphasizes that a classification model’s decisions should be independent of sensitive attributes, such as race or gender, given the true outcome. We define our fairness evaluation metrics as being independent of sensitive attributes. This criterion helps to prevent bias and discrimination by ensuring that the likelihood of a positive outcome, given the model’s decision, does not differ based on sensitive attributes. We measure this using ‘True Positve Rates’ and ‘False Positive Rates’
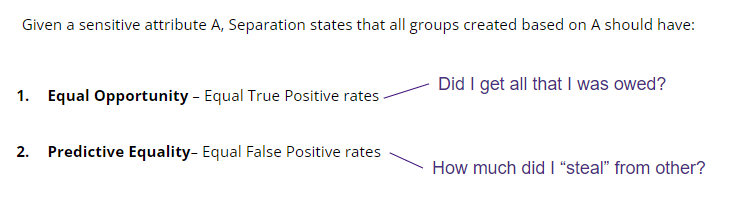
- ‘Overestimation’ and ‘Underestimation’ metrics to include magnitude and direction of errors in flow estimation:
Traditional fairness metrics often focus on overall error rates but may overlook whether a model systematically overestimates or underestimates outcomes for specific groups. By incorporating ‘overestimation’ and ‘underestimation’ metrics, we can gain a more nuanced understanding of a model’s performance and biases, especially not just total errors but also directions of those errors.
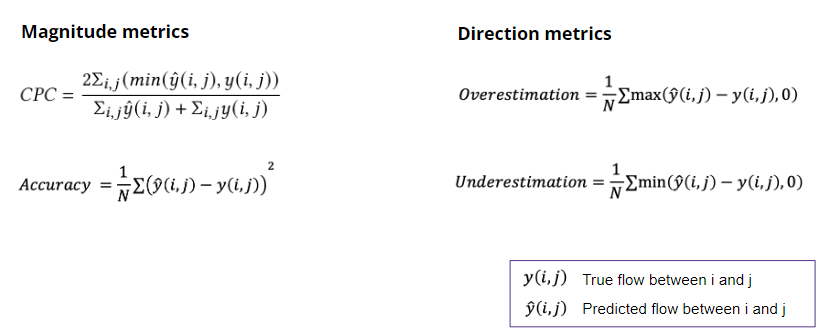